Reduce False Positives in Healthcare Fraud Detection
Part 2 of our series on how the SIU can use artificial intelligence to overcome common challenges. A.I. can reduce the fraud false positive rate to make the most of your limited investigator resources.
Fraud false positives. It’s the single biggest barrier standing in the way of your SIU being able to do more with less. And the traditional approaches to healthcare fraud detection often make this challenge more difficult. Either highly specific rules designed to catch very narrow instances of fraud, or broader anomaly detection that picks up every outlier no matter the intent. The concerns surrounding false positives are real. But with artificial intelligence-powered healthcare fraud detection that features multi-tiered scoring, you can find novel fraud, keep schemes updated, and still focus investigative efforts. Here’s how.
How Do I Find Schemes I Don’t Know About?
For investigators and SIU leaders alike, one of the recurring challenges involves detecting novel fraud. Depending on how you approach this issue, your quest to ensure you don’t miss emerging fraud schemes could lead to a spike in your fraud false positive rate. Let’s look at the differences between attempting to detect novel fraud with querying based on rules versus A.I.
The “pull” approach: Detecting novel fraud with rules
When you know exactly what you’re looking for, rules-based detection can more easily filter out fraud false positives. Highly specific rules yield a high degree of accuracy in the leads you pull. But with that accuracy, you sacrifice some missed opportunities.
So, how do you find what you don’t know to look for? Say you receive a tip on a code or code set that could be problematic. It would create too much over-work and abrasion to investigate every provider that has billed for that code. Not to mention also looking for services not rendered, upcoding and excessive visits.
Rather, to test your theory in the rules-based environment without increasing your false positive rate, you would need:
- A good understanding of the past behavior of your providers, your members, and the schemes you’ve been seeing over the past 18-24 months, along with peer analysis for given specialties
- Ability to establish a baseline to compare the suspected providers and/or claims against. For instance, should you be looking at the trends of a given provider for telehealth, labs, office visits, etc. over the last two years, the last eight months, or the last three months? And, how are you determining peer providers?
- Results of multiple rules related to the theory and the ability to layer those results together to yield likely leads or avenues of further investigation
- Ability to link all rules findings together to achieve big-picture context
The “push” approach: Detecting novel fraud with A.I.
On the other hand, fraud detection based on A.I. does not rely on your discrete inputs or theories to surface schemes – whether known or unknown. Instead, it relies on intelligence within your data to push likely leads to your attention. And, it can do so with relatively little up-front knowledge. But, to address the complexity of healthcare fraud while reducing the number of false positives, many of these systems require massive amounts of labeled fraud case data. As a result, this limited-approach A.I. is impractical for most health plans.
Fraud detection solutions that leverage multiple forms of A.I., ingest multiple types of data inputs, use multiple models to parse the data, create blocks of time windows to explicitly determine when behavior changed, and use multiple scoring mechanisms, can more easily overcome this limitation. The combination of approaches allows for a deeper and wider view of the data, so investigators are served only the most accurate, high target leads.
These solutions – like Pareo Fraud – can better account for the complexity of healthcare fraud even when answering a relatively straightforward question like, “What is a peer provider?” So, accounting for not just geography, specialty, frequency of billing, patient mix, total patient cost of care and network participation. But also:
- Line amounts
- Code billing frequency
- Average daily cost of care
- Recent billing habits versus historical
- Individual provider versus all their patients
- Individual provider versus a single patient
- Single patient versus all their other providers
This level of detail goes into every A.I. model. Layered on top of that is more specific information: impossible days, high dollar amounts, high dollar allowed amounts, high paid amounts, frequency of billing, shadow billing, and more. If it sounds like a lot, it is. Altogether, this multidimensional approach to the data provides context that drastically reduces false positives.
How Do I Keep Schemes Updated?
Related to detecting novel fraud, SIU leaders have a responsibility to keep their detection methods up to date to account for continually evolving healthcare fraud schemes. Creating new rules or looking to your detection tool vendor to release new rules is one option. But this reactive practice may put you further and further behind.
By the time you identify a problem, identify what the rule needs to be and all the codes that need to go with it, implement and test the rule and start running it, the scheme may have changed again. This retrospective approach may prove especially problematic if your organization is focusing on prevention and moving more work prepay.
A.I.-based fraud detection evolves and gets smarter over time. The A.I. models respond to data inputs and investigator review of leads to constantly improve its output. That means, a provider you didn’t suspect yesterday could rise to the level of a lead today based on historical claims data. Without you having to explicitly tell it to look for new schemes, or broadening your search and picking up more fraud false positives. It presents a total picture of your providers and their actions, along with the actions of peer providers and members.
How Do I Focus Investigative Efforts on Likely Leads Instead of Fraud False Positives?
Creating more rules doesn’t necessarily lead to greater accuracy or an increased number of leads. Finding high-dollar claims doesn’t necessarily mean those dollars are actionable or recoverable. And, unfortunately, you can never completely eliminate false positives. But with A.I. you can minimize them and focus your efforts on investigations more likely to yield results.
Configure models specific to your organization
Pareo Fraud uses more than 40 A.I. models to score providers and claims. The results of these models roll up into a SuperScore™ that helps you prioritize potential incidences of fraud that may require additional investigation. The SIU can configure the sensitivity or weighting of these models to account for your health plan’s unique situation.
For instance, your health plan may cover a small geographic area. In that case, the geographic discrepancy – how far members are traveling for care – may be less important. But you may know impossible days is a high-priority metric for your organization. These adjustments ensure the right leads come to your attention.
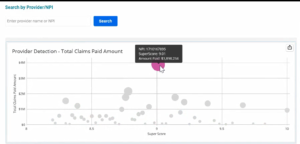
Pareo Fraud highlights those providers with high scores and high-dollar impact.
Drill-down for immediate access to explanations of A.I. results
A.I.-powered fraud detection also can support quickly vetting the legitimacy of likely leads. Review the provider and see, at-a-glance, the explanations for the high score. You can immediately make a determination or go deeper into the associated claims. Filter and search by service ranges, lines of business, claim number, minimum amounts, codes, modifiers, HCPCS, and more. Then, add those claims to an investigation, exclude the provider from detection for a set period of time, or flag as a non-viable lead.
Turn Data into Actionable Information with Pareo Fraud
Individual pieces of information about providers, schemes and code sets won’t help you reduce fraud false positives or detect more fraud. Applications of A.I. put this information together to show you useful intelligence you can use to surface insights and make decisions. Find trends and schemes, reduce false positives, and locate spikes before they turn into big losses.
If your SIU is charged with doing more with less, Pareo Fraud can reduce fraud false positives and help you make the most of your valuable expert resources:
- Automated initial detection and triage through A.I. models based on deep learning
- Extended capability to identify relationships within the data
- Highly specific results rendered through multi-tiered scoring
- Improved intelligence and efficiency through integration with payment integrity
How can A.I. + Domain Expertise help your health plan scale FWA operations?
Most health plans are discovering the hard way that their familiar, legacy fraud solutions aren’t helping them combat false positives, mitigate provider abrasion, work seamlessly with payment integrity functions, or make the most of limited resources. Now that advanced solutions that harness the power of artificial intelligence have emerged for fraud detection, is it the right solution for your organization to hit escalating fraud recovery goals and prove the value of your SIU team’s efforts?